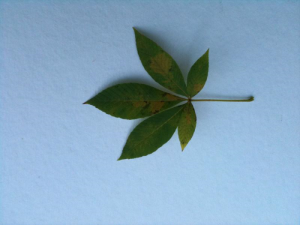
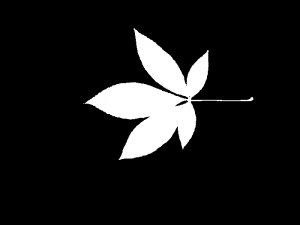
Work Abstract
We propose a robust and accurate method for segmenting specular objects acquired under loosely controlled conditions. We focus here on leaves because leaf segmentation plays a crucial role for plant identification, and accurately capturing the local boundary structures is critical for the success of the recognition. Popular techniques are based on Expectation-Maximization and estimate the color distributions of the background and foreground pixels of the input image. As we show, such approaches suffer in presence of shadows and reflections thus leading to inaccurate detected shapes. Classification-based methods are more robust because they can exploit prior information, however they do not adapt to the specific capturing conditions for the input image. Methods with regularization terms are prone to smooth the segments boundaries, which is undesirable. In this paper, we show we can get the best of the EM-based and classification-based methods by first segmenting the pixels around the leaf boundary, and use them to initialize the color distributions of an EM optimization. We show that this simple approach results in a robust and accurate method.
Dataset
We release hand-drawn ground truth segmentations for 300 different leaf images selected from the publicly available Leafsnap Field dataset [1].
Ground truth images result to be very useful to determine accuracy of a given segmentation method. In our paper [2] we chose and used Field images since they are taken in loosely controlled and non-uniform conditions, thus producing challenging conditions for automatic segmentation.
In this dataset two different subsets are included:
- leaves_testing_set_1: 150 leaf images for which state-of-the-art methods produce already faithful segmentation results;
- leaves_testing_set_2: 150 leaf images for which state-of-the-art methods partially or totally fail.
If you use this dataset, please cite the following papers:
[1] N. Kumar, P. N. Belhumeur, A. Biswas, D. W. Jacobs, W. J. Kress, I. C. Lopez, J. V. B. Soares. Leafsnap: A Computer Vision System for Automatic Plant Species Identification. ECCV 2012. (http://leafsnap.com/)
[2] Simone Buoncompagni, Dario Maio and Vincent Lepetit. Leaf Segmentation under Loosely Controlled Conditions. In Xianghua Xie, Mark W. Jones, and Gary K. L. Tam, editors, Proceedings of the British Machine Vision Conference (BMVC), pages 133.1-133.12. BMVA Press, September 2015. [PDF] [SUPPL. MATERIAL] [Citation]
To see correspondences between leaves and species, please refer to http://leafsnap.com/dataset/
For questions, comments and suggestions, please contact simone.buoncompagni2@unibo.it
Dataset download: CLICK HERE!